Should someone’s marital status impact their car insurance premium? How about if a company restructures and the policyholder’s employment status changes to unemployed? What about their credit card score? What if they are vulnerable due to having a low income? Age? Race? What about a factor that can have a close correlation to race, such as the area someone lives in?
The public discourse regarding what is and is not fair to use in pricing is gaining momentum. There is a difficult balance between actuarial experience and fairness. The use of some characteristics in pricing decisions can cause unfairness or perceived unfairness. As markets evolve, aided by artificial intelligence, there is a risk that inequalities may be unfairly perpetuated and amplified through underwriting. What rating factors should insurers be restricted from using to discriminate between risks due to social justice considerations? Is it even appropriate to use insurance as a social good to cross subsidise risks?
What factors can insurers legally use?
Some factors can legally be used for price discrimination, which may not necessarily be perceived as fair. For example, people on lower incomes can in some instances present a higher risk to insurers due to a variety of factors, some of which may be considered outside their control. People on low income may also be vulnerable and less able to afford higher insurance premiums. They are also less likely to be financially resilient to some risks and unexpected shocks, which insurance can provide protection against. The “poverty premium” is a term often used to describe a situation where those on low incomes pay extra for essential services such as credit, energy and insurance. Research has found that insurance is one of the biggest contributors to the "poverty premium" in the United Kingdom.
The Equality Act 2010 sets out a number of protected characteristics such as age, disability, gender reassignment, marriage and civil partnership, pregnancy and maternity, race, religion or belief, sex and sexual orientation. In general, insurers must not discriminate against a person because of a protected characteristic, including in relation to the premium for the product. However, actuarially justified discrimination based on some protected characteristics is currently lawful where a relationship between the characteristic and loss propensity is established. This is known as the ‘actuarial defence’. For example, age can be used as part of a risk assessment if the information used is relevant and comes from a source on which it is reasonable to rely. Relevance must be actuarially sound. Untested assumptions, stereotypes and generalisations in respect of age are not allowed.
A number of rating factors can be linked to economic status and cause a "poverty premium" such as age, credit score, home address, payment method and employment status. Protected characteristics such as race, sex and disability can also correlate with poverty attributes. Even where certain characteristics are not used, there is a risk of algorithmic proxies to those characteristics being used in pricing. On the other hand, removing some rating factors from insurance pricing could cause many customers to experience price increases as the cost of higher risk insureds is spread across all insureds.
Consumers with protected characteristics are more likely to experience the "poverty premium". Protected characteristics such as race, sex and disability can correlate with poverty attributes and result in different prices for those groups. It is never acceptable for information regarding race to be used in assessing risk. However, Bangladeshi, Pakistani and Black people are disproportionately likely to live in deprived areas, which can impact the cost of insurance premiums. Artificial intelligence may exacerbate the risk of algorithmic proxies to protected characteristics being used in pricing, as it may establish previously unknown patterns and increase the complexity of models. Research has found that people from Black, Asian and other ethnic minority households, lone parents, and disabled people were less likely to hold any insurance. Going without is often the alternative to paying the "poverty premium".
Currently the default approach is to exclude protected characteristics from model specification, so that the model is unaware of them. However, this can result in discrimination by proxy when unfair factors correlate with ‘legitimate factors’. This may disadvantage people with protected characteristics or vulnerability. An alternative to this is explicit equalisation, where all factors – whether fair or not - are included in the model specification. The discriminatory effects are then removed by averaging outcomes across the unfair factors. However, there may also be legal issues with this approach, and difficulties in obtaining all the data necessary to make it work. It can also result in uncompetitive pricing.
Unfair price discrimination in essential products, such as home insurance and car insurance (where it is a legal requirement), is considered by some as a greater concern than in non-essential products. Where insurance is mandatory or essentially mandatory it can be seen as more of a social good and less of an economic commodity.
People living in high crime rate areas are likely to be charged higher home insurance premiums because of a greater risk of home burglary. However, they may be less able to reduce their risk due to factors outside their control. For example, because they do not have the financial means to do so. Some may consider this fair, others may not and still others may consider it unfair, but outside the responsibility of the insurance market as just a reflection of wider inequalities in society.
Increasingly individualised risk pricing
Artificial intelligence will increase the importance of issues regarding what characteristics can be used and the identification of algorithmic proxies to banned characteristics.
Artificial intelligence is accelerating the trend towards more granular, precise pricing based on an individual’s specific rating factors. Whist individualised risk pricing can incentivise positive behaviours that reduce risk and lead to more accurate pricing, it can also create negative outcomes. This can be seen as especially unfair where pricing is based on factors which people have little or no control over. Those with lower risk may enjoy lower premiums, however higher risks may pay more. Some may not be able to afford essential insurance products at all, leading to serious issues.
“Premiums are being set for smaller and smaller subgroups of the population, and ultimately for individuals. This is resulting in losers as well as winners.”
https://actuaries.org.uk/media/31hbykda/campaign-recommendations-april-2021.pdf
In Canada, two different systems of motor insurance operate. Some provinces allow risk pricing and others have pricing restrictions, which mean that everyone pays the same basic insurance premium. In the provinces where risk pricing is allowed, the chance of being involved in a fatal road crash is almost 20% lower than in the more heavily regulated provinces. (https://www.abi.org.uk/globalassets/sitecore/files/documents/publications/public/migrated/how-insurance-works/abi-insurance-in-the-uk_the-benefits-of-pricing-risk.pdf).
Risk pricing can have the benefit of leading to safer behaviour.
AI could also open the door to hyper personalised risk scores. This could allow premiums to be based on people’s actual behaviour, such as their exercise regime, and not just the risk profile of a category to which they belong, such as their age group or postcode. However, hyper personalisation will not only apply to factors that people can control. (https://www.gov.uk/government/publications/cdei-publishes-its-first-series-of-three-snapshot-papers-ethical-issues-in-ai/snapshot-paper-ai-and-personal-insurance#how-might-ai-change-insurance)
There has also been a rapid growth in information regarding genetic propensity to various health conditions. Insurers have an interest in assessing the level of risk to be covered. How much of this personal information should insurers be allowed access to? Insurers may be concerned about adverse selection – the tendency for individuals with knowledge of their genetically based health risks to buy life or health insurance products. This may expose insurers to a greater than expected probability of claims. Does this make it reasonable for insurers to seek genetic information, which individuals have no control over, from policyholders?
Some countries allow insurers to ask for disclosure of results of previous genetic tests, to request new tests, and to take this information into account in setting premiums. However, others do not allow insurers to do any of these things. In the UK there is a Code on Genetic Testing and Insurance between the government and the Association of British Insurers, although this is a voluntary agreement. It commits insurers signed up to the code to never require or pressure any applicant to undertake a predictive or diagnostic genetic test. Under the Code, insurers may only ask applicants to disclose and consider the result of a predictive genetic test in a small number of situations. Huntington’s disease is currently the only condition included, and only in applications for life insurance cover over £500,000. (https://assets.publishing.service.gov.uk/media/5bd08dd1ed915d78af510220/code-on-genetic-testing-and-insurance.pdf)
What does this mean for UK insurers?
The debate over what are ‘fair’ factors to use in insurance pricing and underwriting may accelerate with the use of artificial intelligence. Artificial intelligence could put the ‘actuarial defence’ to the test and cause the relationship between pricing according to risk and discrimination to be re-examined and subject to further regulatory scrutiny.
Insurers need to be able to explain how their pricing systems and practices comply with their obligations under the Equality Act 2010. This remains highly relevant when artificial intelligence is used.
‘Price and value’ is one of the four outcomes that firms need to assess under the Consumer Duty. Differential pricing for different groups of consumers creates considerations for firms’ fair value assessments, which are required by the FCA. There are a variety of ways to segment customers, look at differential outcomes for consumers and tailor analysis. Firms must also have regard to consumers with characteristics of vulnerability and consider and facilitate acceptable outcomes for vulnerable customers. The FCA says:
“Our price and value outcome rules do not require firms to charge all customers the same amount, or to make the same level of profit from all customers.” (https://www.fca.org.uk/publications/good-and-poor-practice/consumer-duty-findings-our-review-fair-value-frameworks).
That said, providing fair value to different groups of customers is central to the FCA’s rules. Firms need to demonstrate how each group of customers receives fair value, even though they can be differentially priced. (https://www.fca.org.uk/publication/discussion/dp18-09.pdf, https://www.fca.org.uk/publication/thematic-reviews/tr18-4.pdf)
The Institute and Faculty of Actuaries has recommended that the government determines an appropriate minimum level of insurance protection needed by all, including low income families, to enable financial resilience to specific risks and unexpected shocks. It acknowledges that as insurers are commercial organisations, they need to manage their business by reflecting risk in pricing. It therefore sees the government as having the key role in identifying and protecting vulnerable groups. (campaign-recommendations-april-2021.pdf (actuaries.org.uk))
There is a trade-off between risk pooling with uniform pricing at one extreme, and cross subsidisation and individualisation at the other. Hyper individualised risk pricing can lead to higher prices for some customers, who are often the most vulnerable and low income. Some contend that consumers should not be penalised for factors that are outside of their control, however there are differing views on what is outside a person’s control. Artificial intelligence adds a further dimension to this debate.
Contents
Key contact
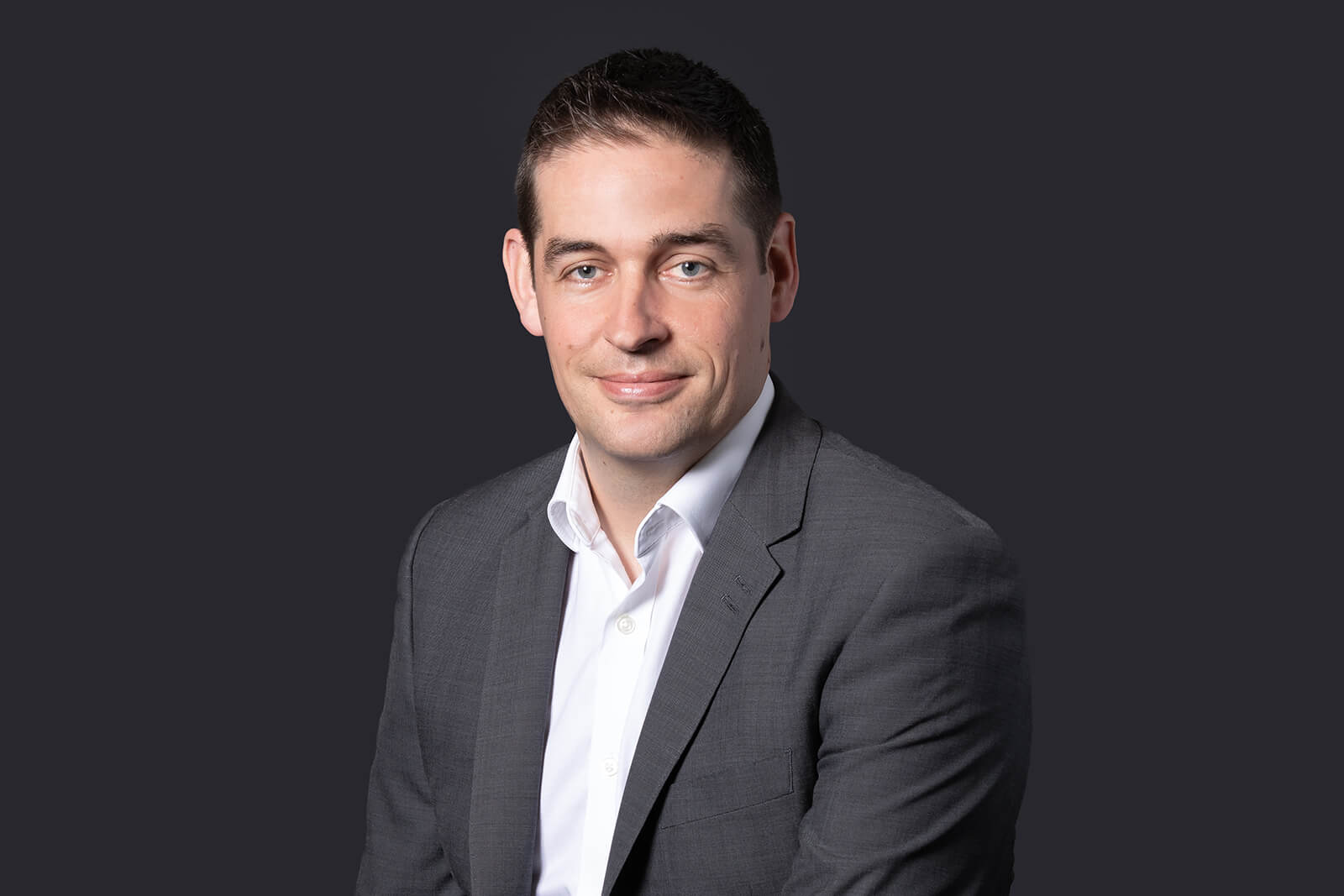
Tim Johnson
Partner
tim.johnson@brownejacobson.com
+44 (0)115 976 6557